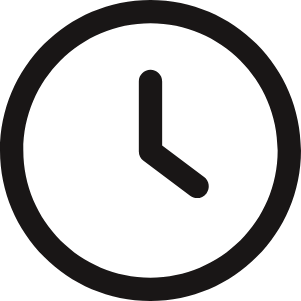
Neurocle is a deep learning vision software company that hopes to enrich the world with their deep learning technology, striving to catalyse success for clients and partners to embark on an innovative journey with their releases of products -Neuro-T and Neuro-R.
Guide to using Neurocle’s Deep Learning Vision Software -Neuro-T and Neuro-R.
Anyone can create and utilize deep learning vision models with Neuro-T and Neuro-R. Neuro-T automatically trains images and builds them a deep learning model. Neuro-R enables users to run real-time image analysis across a variety of areas including manufacturing, logistics and medical field with models customised by users themselves.
Creating a deep learning vision model, in Neuro-T, first upload your training images and go to the data tab, select the model data type that best fits your image analysis objectives. User can create multiple types of models with one data set. The model types include classification, segmentation, object detection, OCR and anomaly detection. Classification refers to sorting of images to different categories. Segmentation is recognising the shapes and locations of objects within images. Objection Detection, distinguishing the class and location of objects within images. OCR detects and extracts characters from images. Lastly, Anomaly Detection, identifies outliers that deviate from the majority of the data.
If there is an area that needs to be focused or excluded, for an accurate image training, specify it and designate a class to each image, splitting the images into training and model performance testing sets. In the train tab, check the label information, adjust the settings, and start training.
Neurocle’s auto deep learning algorithm automatically optimises the images’ parameters. Check the created model in the evaluation tab, user can visualise the model performance by checking the test images as well as writing a report about the results.
Finally, export deep learning model as a .net file. By using the information from the report, the user can write a research paper and build a smart factory by integrating deep learning models into his equipment using Neuro-R. -Apply a generated deep learning vision model for visual inspection.
Create the utilized optimal deep learning models with Neuro-T and Neuro-R with the automatic deep learning algorithm, making deep learning vision technology more accessible.
![]() |
![]() |
Unique Features of Neuro-T and Neuro-R
Neuro-T and Neuro-R includes unique features such as 1. Auto Deep Learning Algorithm, 2. Fast Retraining Feature, 3. Server-Client Based Data Management System, 4. Fast Inference Time and 5. Supports Diverse Platforms.
- 1. Auto Deep Learning Algorithm
To create optimized deep learning models in one simple train. Through Auto Deep Learning Algorithm, even non-experts can easily create high-performance models and save valuable time and resources compared to traditional models.
Generally, models require a long lengthy process to complete and reach the final model stage. They must go through DL Architecture to Hyperparameter to Data Augmentation and repeated trainings before arriving at the final model. However, with Neuro-T, a simple click of auto optimization through auto deep learning can achieve a high-performance model.
- 2. Fast Retraining Feature
Guarantees performance and maintenance of existing DL models. Through Fast Retraining, models can be more quickly and accurately adapted to changes in inspection equipment or environment than general retraining or transfer learning methods.
General retraining requires several trails and prolonged time and collection of all train data and even prolonged training time. Whereas, transfer learning, additional data to be collected and short training time does not guarantee high performance due to the lack of data. With Neuro-T, all the collected train data adding on short training time can achieve high accuracy in short training time, fast retraining.
- 3. Server-Cilent Based Data Management System
Collaborate with a single license. Neuro-T’s server-client architecture makes it possible to effectively collaborate with users without physical, spatial and device constraints. Comparing other general project management structures to Neuro-T’s project management structure, general project management structures consist of high device dependency and requires purchasing a license for each device which means local PCs cannot be connected to other local PCs with the same license. However, Neuro-T’s project management structure is possible to collaborate by accessing to one software from multiple devices. This means that a local server PC can be connected to not only another local PC but as well as Client PC, Client Laptop and Client Tablet PC, bringing way more convenience and accessibility compared to general project management structures.
- 4. Fast Inference Time
Provides high inference speed for sites where processing time is crucial. Inference speed can be freely adjusted as needed and enables fast inference speed to real-time inspection. The inference time at level one is specialised for fast speed which may be faster during batch processing. For example, 1.4ms based on Object Detection 8 Batch. At Level 1, classification processing time is 1.3 to 1.7ms, segmentation is 2.2 to 2.3ms and object detection is 3.5 to 4.0ms. If level 1’s speed is too fast, level 3 does not have any speed limit. For classification, processing time at level 3 is 1.3 to 3.8ms, segmentation is 2.2 to 4.5ms and object detection is 3.5 to 6.8ms.
- 5. Supports Diverse Platforms
Customise to any device regardless of its specification. Using Neuro-R, apply your model to any platform such as embedded processors, CPUs, and GPUs that best suits your industry. Neuro-R can be used on various platforms ranges from light industries such as consumers good crops with platforms in embedded-based like jetson to heavy industries such as manufacturing, for example semiconductor, display and security image using PC-based multi-GPU.